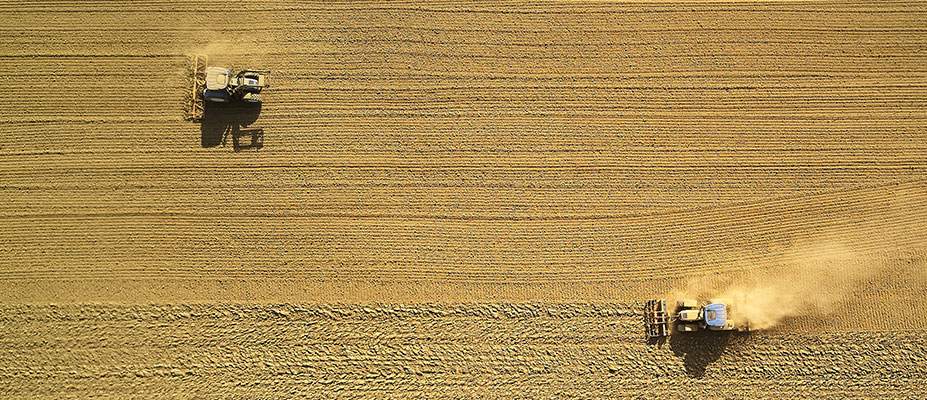
Predicting crop yield within the growing season at sub-paddock scale: a big data approach
PhD Candidate: Robert Clark
Supervisors: Assoc Prof Peter Dahlhaus, Dr Nathan Robinson, and Dr Elizabeth Morse-McNabb (Department of Jobs Precincts and Regions).
Research Overview
The availability of accurate and timely predictions of crop yield within the growing season would be of enormous benefit to the grains industry in Australia. Such a development would allow state governments and other industry level providers to optimise the management of infrastructure and transport systems, marketing organisations to negotiate contracts with more certainty, service providers to manage their workforce and plant more efficiently and farmers to make crop management decisions and set up forward contracts with more confidence.
The aim of this research is to develop a prediction algorithm that will produce timely, robust, crop yield estimates at sub-paddock scale within the growing season for the main commercial broad acre crops. The algorithm will need to account for variation caused by the different climatic zones, soil types and farming systems that occur across a variety of cropping regions and across different seasons. The crop yield estimates will be based on a range of publicly available data sets including satellite imagery, landscape metrics, soil moisture estimates and meteorological data. Yield estimates based on freely available open data will be cost effective to produce in the future.
The research will apply a selection of multi-variate statistical analysis and machine learning techniques to the remotely sensed imagery, soil data, climate data and landscape metrics. Yield data collected by farmers and service providers at harvest will be used to develop and calibrate the prediction algorithms and test the accuracy of the estimates. For that reason it will be essential to develop strong links with farmers, contractors, growers groups and other researchers. Historic yield data that overlaps with appropriate satellite imagery and other data will be used to calibrate and test the estimates across multiple seasons to make the algorithm more robust. It is intended to utilise eResearch tools and techniques to identify potential sources of legacy ground truth data, analyse and process the data and to publish any new data sets developed in the course of the research project.
This research aligns closely with GRDC priorities on extracting greater value from spatially referenced data which is already being collected and identifying ‘the yield gap’ and areas where tactical agronomy can be deployed in response to manageable factors impacting production.